Since the public release of ChatGPT just over a year ago, AI has driven technology investment, with some $27B being deployed to companies leveraging the latest developments in machine learning
So what? Space engineers are no strangers to AI writ large—who do you think is flying the Dragon spacecraft or landing the Falcon 9’s booster?—but the latest developments promise immediate impact for companies collecting sensor data in space.
Background: The innovation behind ChatGPT, an advancement in learning models based on the human brain called transformer neural networks, are only as valuable as the data used to train them. Last year, Salesforce CEO Marc Benioff told investors that AI “customer value will only be provided by companies who have the data.” Deloitte, the global consulting firm, argues that generative AI will be transformative for firms that collect unique data sets with satellites, which include Maxar, Planet Labs, Spire, Capella, ICEye and many others.
Planet Labs, a space data firm operating roughly 200 satellites in orbit, has long premised its business model on using machine learning to divine insights from its daily scans of the Earth. Payload spoke to Nate Gonzalez, Planet’s head of product, to understand what the last year of AI hype means for earth observation companies like his.
This transcript has been edited for length and clarity.
Have you noticed a change in the vibe of the tech community in the last year because of these advances in AI?
Yeah, which is funny cause I think that trying to pull apart AI from [Machine Learning] and [Large Language Models], it is kind of a veneer. The reality is everything is now powered by AI or everything has a flavor of it because like that’s where the dollars are coming from and it’s a hard market.
We’re in that cycle, and what I take a lot of comfort in is that our team knows it all really well. We’ve been doing it for a long time. We have experts in their fields of how to do these operations, and now there is a new understanding of where that field has gone that they can jump to very quickly, and we can pivot on that and create real product and value there. But it is funny to see how many folks are now AI experts on their LinkedIn
What has changed for Planet, which has been training models on its data to do things like detect roads, buildings and ships since 2019?
It’s not fundamentally different, but it’s accelerated some of the core work. It’s different methodologies of machine learning—it’s built on transformer networks, which is a different methodology of machine learning that is just vastly more powerful for specific applications that we’re starting to see. That’s where we’ve jumped into the future two, three, four years. When I came to the role [in Aug. 2021], I wrote a two year strategy, looked at what was on the horizon, and we’re beyond that.
What’s an example of how transformer models—the advancement in neural networks underlying tools like ChatGPT—work for Planet?
If I looked at the roadmap 18 months ago and we’re thinking about,when are we gonna be able to sit down and input text of, “I would really like to monitor this county in Ireland to tell me when farmers are harvesting their crops, and I would like an alert on that every time a threshold is tripped over X.”
It was a trickier problem—okay, we have all the data, now we can go run and train models and we’re gonna have to be very specific. [But] just leveraging the transformer network model, what’s happening with generative, allows you to search very quickly. And if you think about the depth of our archive, we have more than 50 petabytes of storage.
[When] a customer tells us they want to go access it, we can then run roughly 10x transformations on any bit that sits there….so, we get 500 petabytes of different data assets that can kind of be created and leveraged. It’s like there’s just this mountain of data to be able to sift through and you start getting into your embeddings work. It allows you to find patterns much more quickly than what you’re necessarily looking for. And it allows you to have that chat interface just be almost like, just the front end to a deeper ML interdicted search experience.
We’ve seen some impressive demonstrations; Planet partnered with Synthetic.AI to find the origins of 2023’s Chinese spy balloon, and at an event last year Planet tracked down the “bouncy castles” covering Chinese missile silos based on a Google image search.
That’s what we’re uniquely well-positioned to do: you found the thing, let’s help you analyze that, let’s help you monitor it over time. Right now there’s still a back and forth between Planet and a partner and the customer to say, you care about this specific thing, the partner will help find it or identify or train some of those models very quickly. We will then find that in the data, serve your results, and allow you to monitor that going forward.
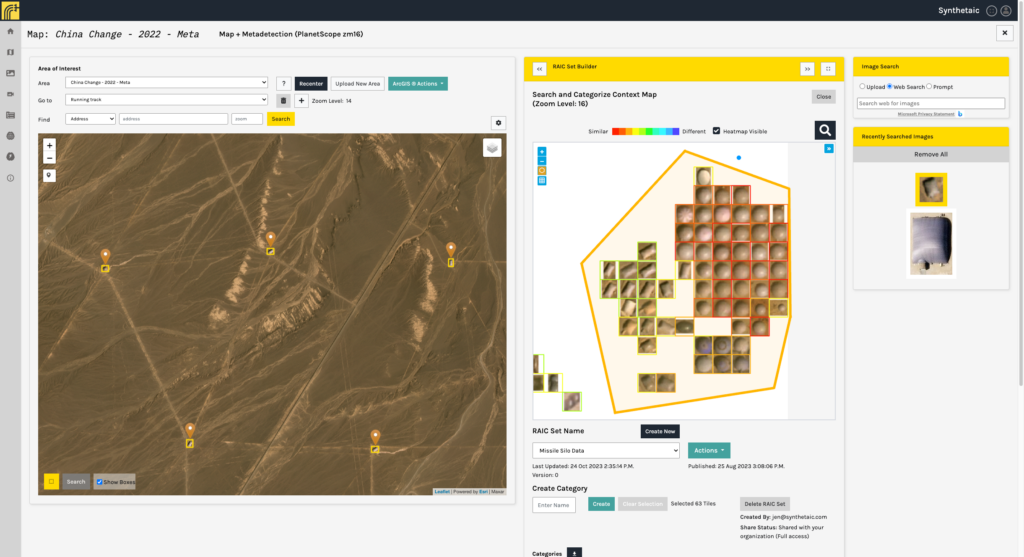
Is it as simple as plugging a generic model into Planet’s data?
It’s hard for Planet to think about training models—generic AI models or ML models in geospatial are not that valuable because a lot of times they are very specific to the use case of the individual customer. That’s where working with partners and having infrastructure that allows partners to work and build with us is really valuable, because we have customers with all kinds of different demands and use cases. If you care about harvesting in the northern hemisphere versus the southern hemisphere, the markers that you’re looking at from the data are slightly different just given the growing seasons and the type of crops.
What about the dreaded issue of AI hallucinations, or errors in models more broadly?
Almost invariably there’s a human in the loop in the training phase. Even in the balloon instances it’s: how are we gonna potentially find a balloon? Draw a rough picture of it, see if we can find the thing and then see how many instances we find and then have a couple of people validate like, yep, that’s actually what we are looking for.
That’s also why the answer is not necessarily to go hire an army of people, because you still have to be mindful of how much time do we want to spend training on something specific for a client versus a partner?
Planet’s data set seems obviously valuable to train machine learning models—is it tempting to license that to large AI companies?
We have a unique set of data that does not exist elsewhere, so we want to be prudent in how we use that, because if you fully open it up and somebody trains a model where they no longer need your observations or they can take some other observation and it’s good enough—you don’t want to disintermediate yourself.
We are partnering liberally because we want to get the value out of the data. The most important thing for customers that we’re working with, who are driving decisions around policies that we care about, is how do we get them value out of the data quickly? I will partner all day long to go do that, to make sure that we’re faster in getting answers for customers.
That’s different than, hey, let’s just monetize the fact that we have this data spigot and turn it on for anybody. Because the reality is that we are running an enterprise sales business where we sell a SaaS product to large civil governments and agricultural groups and others that are getting value from that.